The Power of a Data Labelling Tool in Today's Business Landscape
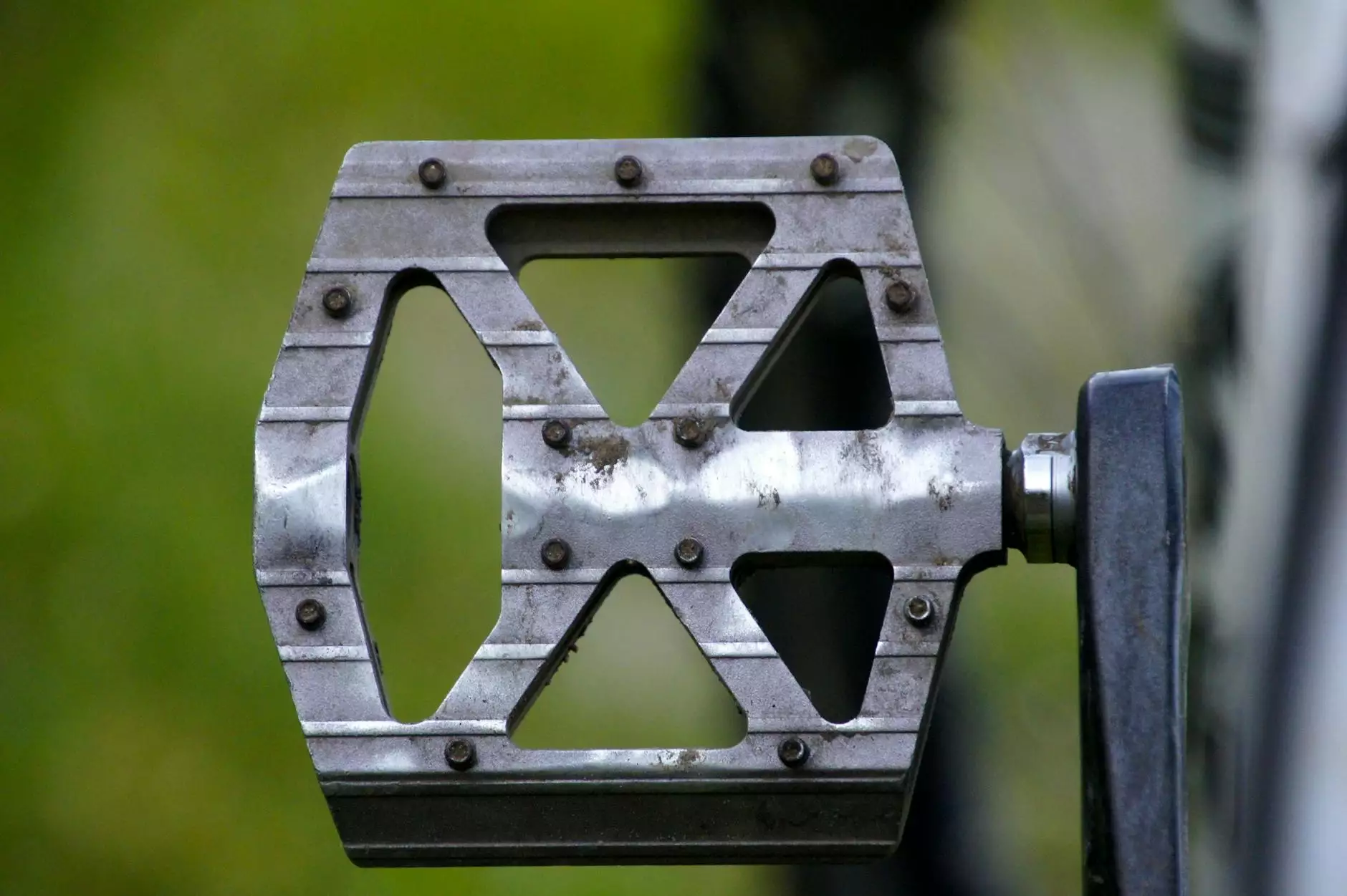
In the fast-paced, technology-driven world we live in, businesses are harnessing the power of artificial intelligence (AI) and machine learning (ML) to stay competitive. A crucial aspect of AI and ML is the quality and precision of data utilized in training models. This is where a data labelling tool comes into play. Using a robust data annotation platform enables companies to not only improve their machine learning models but also to streamline workflows and enhance overall productivity. In this article, we delve deep into the importance of data labelling, highlighting how businesses can benefit from a high-caliber data labelling tool.
Understanding Data Labelling: The Foundation of Machine Learning
Data labelling refers to the process of tagging, annotating, or categorizing data, making it comprehensible for machine learning algorithms. Essentially, it is the building block for training AI models. By providing context to data, businesses can improve the accuracy and effectiveness of their machine learning applications. Whether it's images, text, or audio data, a well-defined labelling process is indispensable.
The Role of a Data Labelling Tool
A data labelling tool acts as a hub for this critical process. It facilitates the systematic annotation of data by providing users with the necessary functionalities to label efficiently and accurately. With the right tool, companies can save time, reduce costs, and enhance the quality of their data, which in turn leads to superior model performance.
Key Features of an Effective Data Labelling Tool
When selecting a data labelling tool, it’s crucial to consider several key features that can significantly influence the annotation process. Here are some essential characteristics:
- User-Friendly Interface: A simple yet powerful interface allows annotators to navigate quickly and label data with ease.
- Support for Multiple Data Types: The tool should allow for various data formats, including images, text, audio, and video.
- Collaborative Annotations: Facilitating teamwork is vital. The ability to collaborate in real-time improves efficiency and consistency in annotations.
- Customizable Workflows: Each project may require different workflows. A good tool should allow customization to fit specific needs.
- Quality Control Mechanisms: Built-in mechanisms for reviewing and validating annotations ensure high-quality data output.
- Integration Capabilities: The ability to integrate with other systems, such as data storage solutions and machine learning platforms, is key for seamless workflows.
Benefits of Utilizing a Data Labelling Tool
Using a data labelling tool offers a myriad of advantages for businesses venturing into AI and machine learning projects. Here’s how these tools can revolutionize your data management:
1. Enhanced Accuracy
The accuracy of machine learning models heavily relies on the quality of the data used for training. A robust data labelling tool minimizes human error through features like standardized labels and review systems, ensuring the output data is as accurate and precise as possible. By employing both automated and manual labelling processes, businesses can achieve significant improvements in data quality.
2. Increased Efficiency
Time is money. Labor-intensive processes can significantly hinder productivity. By implementing a professional data labelling tool, businesses can automate repetitive tasks and streamline workflows. This not only accelerates the annotation process but also frees up valuable human resources to focus on more strategic initiatives.
3. Scalability
As your business grows, the volume of data you work with will likely increase. A data annotation platform allows for scalability in your labelling efforts, accommodating larger datasets without a proportional increase in time or effort. This flexibility is vital for companies looking to evolve in a dynamic market.
4. Cost-Effectiveness
While it may seem that investing in a data labelling tool is an added expense, the long-term savings it can generate are substantial. By reducing errors, accelerating processing times, and improving the overall quality of annotations, businesses can save money on retraining and redeveloping models.
5. Improved Collaboration Among Teams
Incorporating a data labelling tool fosters collaboration. Teams can work together in a centralized platform, sharing insights and feedback easily. This collaboration not only improves the consistency of labels but also stimulates creative problem-solving across departments.
How to Choose the Right Data Labelling Tool for Your Business
Selecting the right data labelling tool is a pivotal decision. Here are some considerations to help guide your choice:
1. Assess Your Specific Needs
What are the types of data you will be annotating? Consider whether you need a tool that accommodates images, text, or video. Understanding your requirements will help narrow your options.
2. Evaluate Usability
The tool you choose should be easy to use for everyone on the team. Look for intuitive design and ample user support to facilitate quick onboarding.
3. Check Customer Reviews and Testimonials
Do your research! Look at user reviews and case studies from other businesses. Real-world experiences can give you insight into the reliability and effectiveness of the tool.
4. Consider Future Needs
As your business evolves, your labelling needs may change. Choose a tool that is adaptable and can grow with your business strategy.
5. Request a Demo or Trial
Whenever possible, request a demonstration of the tool or a trial period. This hands-on experience can assist in determining if it matches your business expectations and workflows.
Integrating Data Labelling Tools into Your Workflow
To maximize the benefits of a data labelling tool, it’s essential to effectively integrate it into your existing workflows. Here are steps you can take to ensure a smooth integration:
1. Define Clear Objectives
Before implementing the tool, establish clear goals for what you aim to achieve. Whether it's improving annotation speed or increasing data accuracy, having defined objectives will guide your use of the tool.
2. Train Your Team
Ensure that your team members are well-trained in using the tool. Provide comprehensive resources and support to facilitate their understanding and usage of the platform.
3. Monitor and Adjust Processes
Once integrated, continuously monitor the performance of the tool and the data annotation processes. Use this insight to make adjustments and improvements over time.
4. Foster a Culture of Feedback
Encouaging teams to provide feedback on the tool's functionality enhances overall efficiency. Create open lines of communication to address any concerns and quickly implement necessary changes.
Case Studies: Successful Implementations of Data Labelling Tools
Understanding the practical applications of a data labelling tool can provide invaluable insight. Here are a few success stories demonstrating the transformative capabilities of such tools:
1. Company A: Optimizing Image Recognition
Company A, a tech startup focusing on image recognition software, utilized a data labelling tool to annotate thousands of images accurately. By leveraging collaborative features, multiple teams contributed to the annotation process, resulting in a 50% reduction in project timelines and a significant improvement in model accuracy.
2. Company B: Advancing Natural Language Processing (NLP)
Company B, which specializes in NLP solutions, adopted a comprehensive data annotation platform to manage vast amounts of textual data. The platform's capabilities for sentiment analysis helped them refine their algorithms, leading to a 40% increase in customer satisfaction due to more accurate text understanding.
3. Company C: Revolutionizing Autonomous Vehicles
Company C, a leader in autonomous vehicle technology, implemented a data labelling tool to annotate video data from various driving scenarios. The efficiency gained from using the platform allowed them to quickly iterate on their machine learning models, expediting their path to market in a highly competitive industry.
The Future of Data Labelling Tools
As technology continues to advance, the capabilities and functionalities of data labelling tools will evolve. Anticipate integration with AI algorithms that can assist in the labelling process, often referred to as "human-in-the-loop" systems, where AI and human annotators work hand-in-hand. These advancements will further enhance the efficiency and accuracy of data annotation, driving business innovation and growth.
Conclusion
Investing in a data labelling tool is not just a step towards better machine learning models; it’s an essential foundation for any business aspiring towards digital transformation. By streamlining the data labelling process, enhancing accuracy, and facilitating collaboration, these tools empower organizations to leverage their data fully. As the demand for high-quality data continues to rise, being at the forefront with advanced data annotation solutions can set businesses apart in a competitive market.
At KeyLabs.ai, we are committed to providing top-of-the-line data annotation platforms tailored to meet your business needs. Discover how we can help you enhance your data management strategies today.